Scaling AI Infrastructure with High-Speed Optical Connectivity
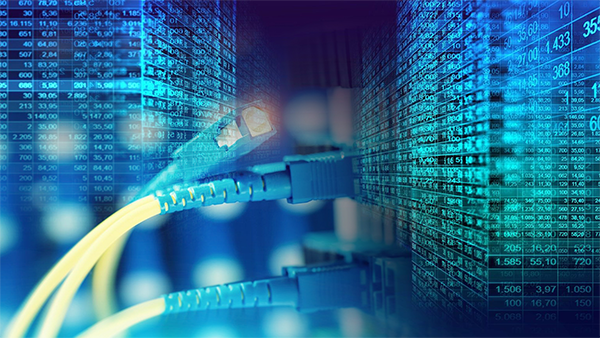
In the world of artificial intelligence (AI), where compute performance often steals the spotlight, there’s an unsung hero working tirelessly behind the scenes. It’s something that connects the dots and propels AI platforms to new frontiers. Welcome to the realm of optical connectivity, where data transfer becomes lightning-fast and AI’s true potential is unleashed. But wait, before you dismiss the idea of optical connectivity as just another technical detail, let’s pause and reflect. Think about it: every breakthrough in AI, every mind-bending innovation, is built on the shoulders of data—massive amounts of it. And to keep up with the insatiable appetite of AI workloads, we need more than just raw compute power. We need a seamless, high-speed highway that allows data to flow freely, powering AI platforms to conquer new challenges.
In this post, I’ll explain the importance of optical connectivity, particularly the role of DSP-based optical connectivity, in driving scalable AI platforms in the cloud. So, buckle up, get ready to embark on a journey where we unlock the true power of AI together.
The Need for Speed in AI Platforms
AI platforms rely on vast amounts of data to train and operate efficiently. The exponential growth of AI model sizes, as shown in the chart below, and the increasing complexity of AI models demand extremely high bandwidth to feed the compute engines in a timely manner.
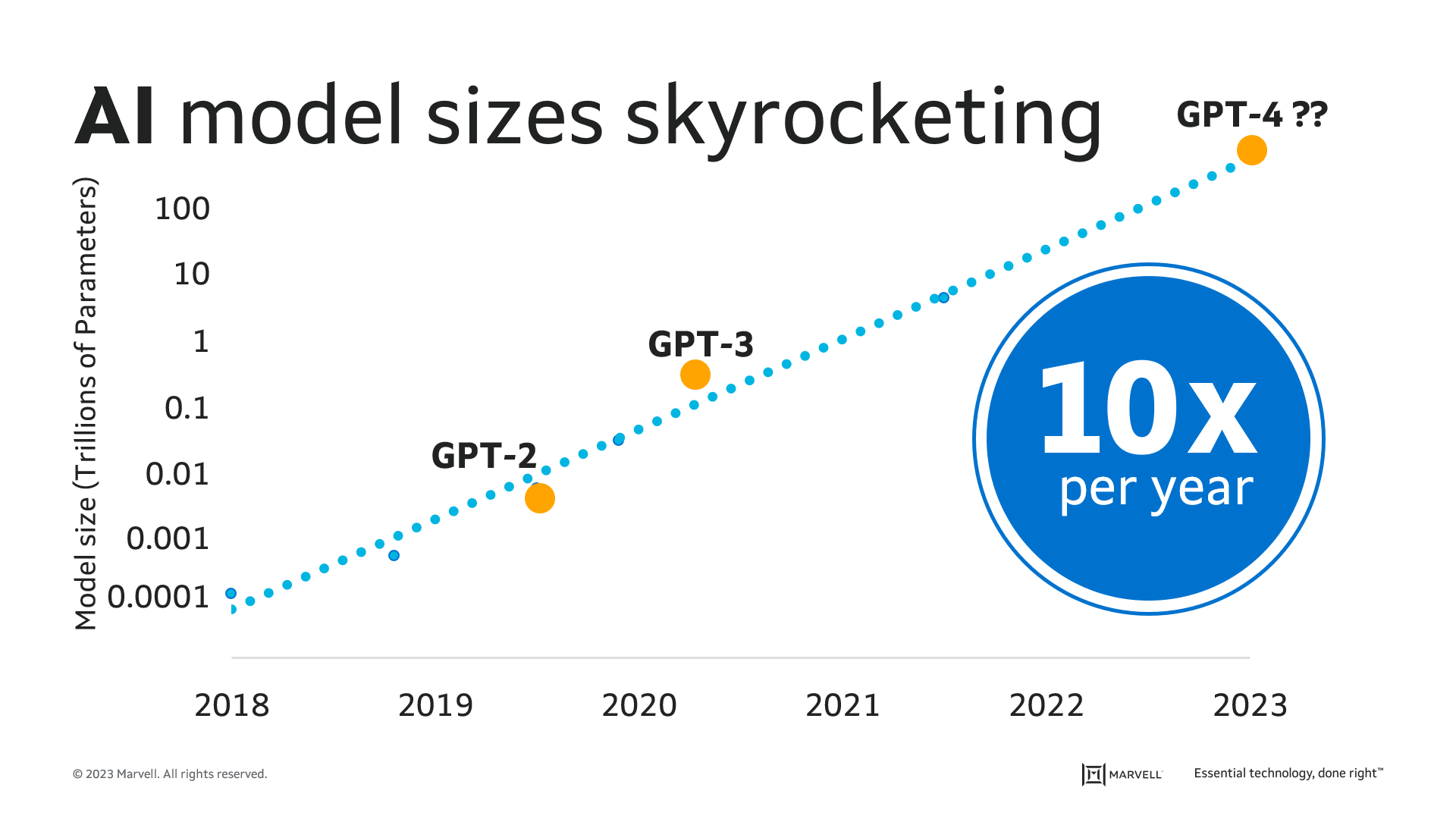
Traditional compute platform architecture vs today’s leading AI platform architectures
To understand the disparities between traditional methods and today’s AI platforms, let’s delve into their respective architectures and performance capabilities. Traditional cloud compute servers are typically constructed with dual socket x86 CPUs, which provide compute performance in the several teraflops range (trillions of floating point operations per second). However, this level of compute performance falls short when it comes to meeting the demanding requirements of AI workloads, such as large language model (LLM) training.
These workloads involve processing massive amounts of data, which, if executed on such traditional architectures, could take months or even years to complete. Recognizing this limitation, AI platforms are designed by clustering purpose-built servers specifically tailored to handle the training of such workloads. The image below showcases one of these specialized servers, which incorporates approximately 8 xPUs or AI accelerator processing units interconnected to deliver several petaflops (quadrillions of floating point operations).
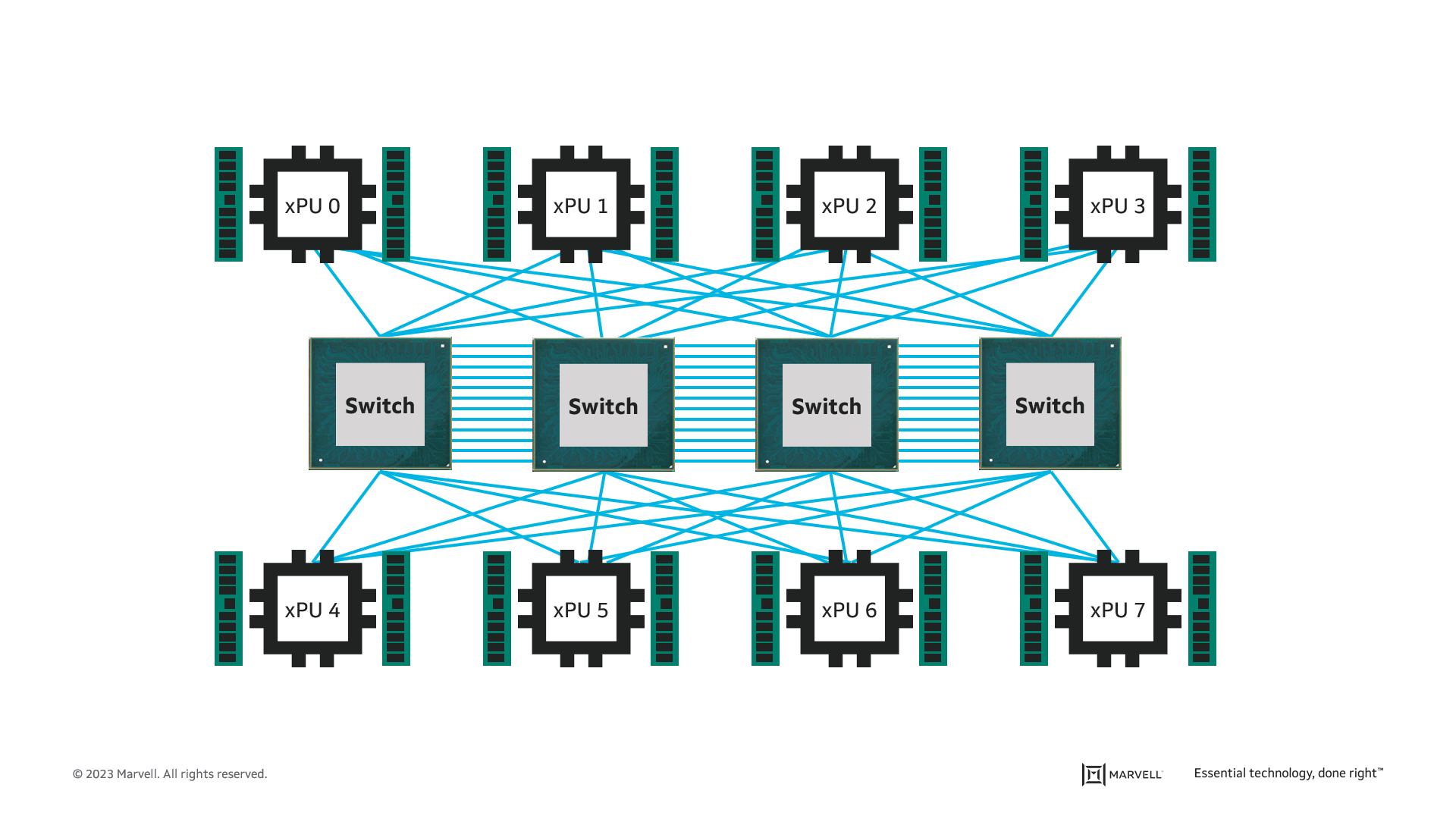
This configuration enables high-performance training and the processing capabilities required by AI workloads.
AI Bandwidth Challenge
Having established the distinctive architecture of AI platforms compared to traditional compute platforms, it is also important to note AI platforms’ massive bandwidth requirements. For instance, each xPU or AI accelerator used in the example AI platform has 3.6Tbps of networking bandwidth resulting in a total bandwidth of nearly 30Tbps per server. In comparison, today’s typical cloud compute server has an average networking bandwidth of 100Gbps. This represents a 300X multiple of bandwidth capacity as compared to today’s typical cloud compute server.
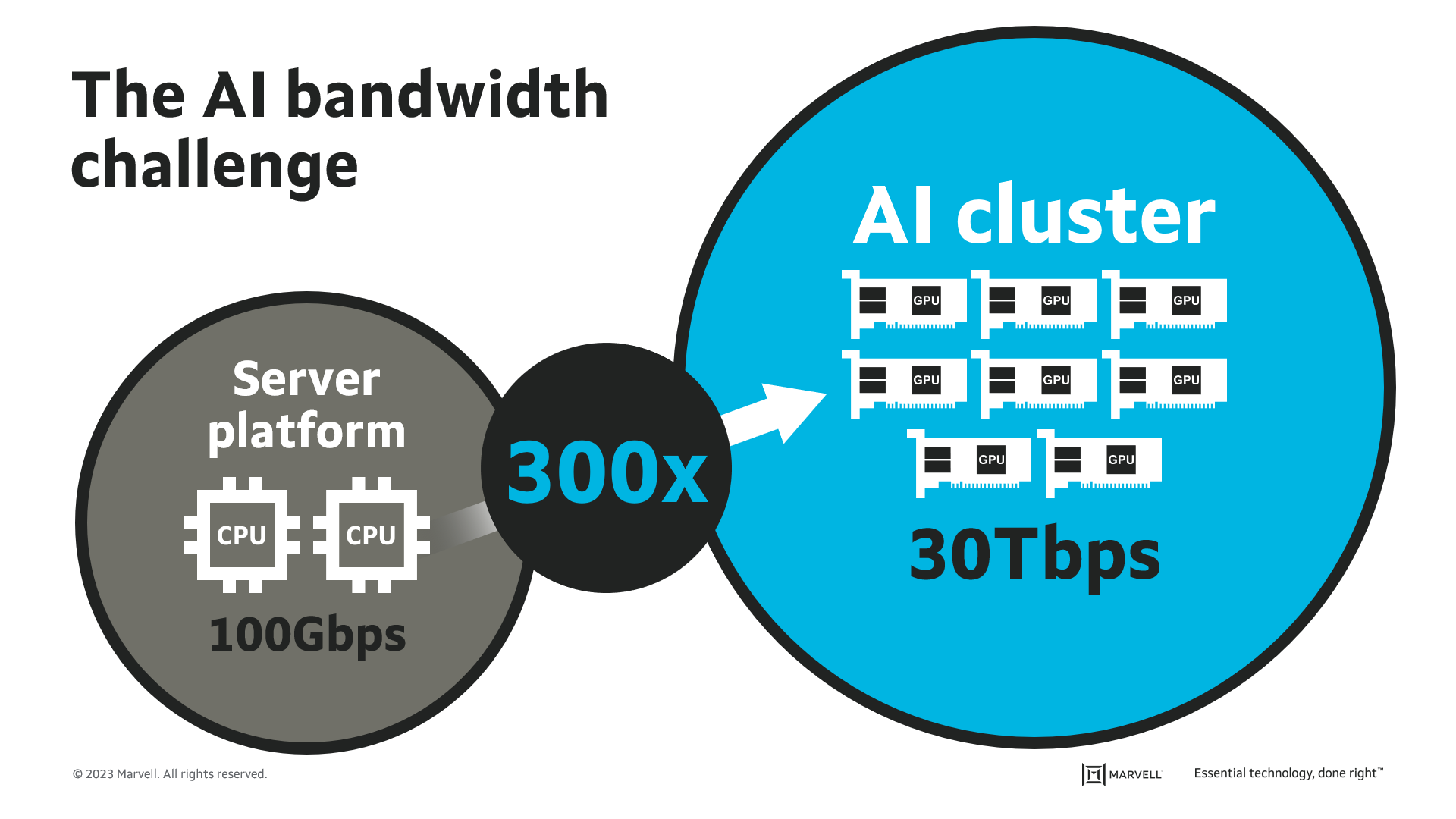
Additionally, a complete AI system is created by connecting 100s or 1000s of the AI clusters shown in the above figure, which is not typical in the case of a traditional server platform. The implication of this stark difference is that to fully leverage the compute capabilities of these AI platforms, it becomes imperative to provide them with data at a rate that matches their consumption capabilities. High-speed connectivity emerges as an essential component for achieving optimal performance in AI platforms. Consequently, leading AI platforms in today’s landscape demand a minimum bandwidth of 800G per connectivity, and even this substantial bandwidth allocation barely scratches the surface of their overall requirements!
AI Platforms Powered by High-Speed PAM4 DSP-based Optical Connectivity
High-speed connectivity is essential for optimal performance in AI platforms. Without it, compute resources would be limited by their inability to efficiently process large training data sets.
When establishing connectivity, there are some drawbacks to traditional hardware. For instance, utilizing traditional Direct Attached Copper (DAC) would introduce limitations. Copper cables suffer from signal degradation over long distances, leading to significant latency and data loss within the AI cluster. As demands of AI platforms continue to increase, the limitations of traditional connectivity options become evident and problematic. It becomes clear that high-speed DSP-based optical connectivity of 800G and beyond is critical for meeting the bandwidth requirements and ensuring seamless data transfer within AI clusters.
In conclusion, optical connectivity is a critical component of AI infrastructures. The exponential growth of AI data sets and model sizes, complex neural networks, and the need for real-time analytics demand high-speed, efficient data transfer. Traditional methods simply cannot keep up with the bandwidth demands of AI workloads. DSP-based optical connectivity powers AI platforms with unmatched performance, low latency, and remarkable bandwidth capacity.
By embracing the power of high-speed optics, AI platforms can reach new heights, unlocking the full potential of AI applications and revolutionizing industries across the board. With the right optical connectivity, the possibilities are limitless.
Are you ready to unleash the true power of AI with high-speed optics? Join the revolution and propel your AI infrastructure to unprecedented levels of performance and efficiency.
This blog contains forward-looking statements within the meaning of the federal securities laws that involve risks and uncertainties. Forward-looking statements include, without limitation, any statement that may predict, forecast, indicate or imply future events or achievements. Actual events or results may differ materially from those contemplated in this blog. Forward-looking statements are only predictions and are subject to risks, uncertainties and assumptions that are difficult to predict, including those described in the “Risk Factors” section of our Annual Reports on Form 10-K, Quarterly Reports on Form 10-Q and other documents filed by us from time to time with the SEC. Forward-looking statements speak only as of the date they are made. Readers are cautioned not to put undue reliance on forward-looking statements, and no person assumes any obligation to update or revise any such forward-looking statements, whether as a result of new information, future events or otherwise.
Tags: AI accelerator processing units, AL Cluster, DSP, DSP based Optical Connectivity, hig speed optics, high speed optical connectivity, Optical Connectivity, PAM4 DSP, PAM4 DSP-based Optical Connectivity